Feature Analysis and Recognition of Induced Uranium Components Fission Signal Based on BP Neural Network
-
摘要: 在对诱发铀部件裂变信号的测量原理及特点分析的基础上,开展了基于BP神经网络的诱发铀部件裂变时间关联信号特征参量分析处理的研究工作。 采用无偏估计方法, 计算信号的自相关函数和互相关函数, 再利用比较法和导数法两种特征量提取方法, 提取出不同状态下裂变信号的特征参量, 借助于BP神经网络模式识别应用原理进行训练和预测。 理论分析和研究结果表明: 基于比较法和导数法获得的特征参量能较好地反映诱发铀部件裂变信号的特征; 用BP神经网络对裂变信号进行模式识别, 取得了较高的正确率, 验证了此方法的有效性和合理性。 The paper presents feature parameter analysis and processing in fission timedependent signal of induced uranium components based on BPNeural Networks through the analysis of the measuring principle and signal characteristics of induced uranium components fission signal. The auto correlation functions and cross correlation functions are calculated by using unbiased estimate, and then the feature parameters of fission signal in different status are extracted by using feature abstraction method, comparative method and derivative method, and then applied to training and prediction by means of BPneural networks based on pattern recognition. Theoretical analysis and the results show that, it is effective to obtain feature parameters of induced uranium component fission signal via comparative method and derivative method. UsingBP neural network to recognize patter of fission signal, we got good results that verified the effectiveness and reasonability of the method.Abstract: The paper presents feature parameter analysis and processing in fission timedependent signal of induced uranium components based on BPNeural Networks through the analysis of the measuring principle and signal characteristics of induced uranium components fission signal. The auto correlation functions and cross correlation functions are calculated by using unbiased estimate, and then the feature parameters of fission signal in different status are extracted by using feature abstraction method, comparative method and derivative method, and then applied to training and prediction by means of BPneural networks based on pattern recognition. Theoretical analysis and the results show that, it is effective to obtain feature parameters of induced uranium component fission signal via comparative method and derivative method. UsingBP neural network to recognize patter of fission signal, we got good results that verified the effectiveness and reasonability of the method.
-
Key words:
- uranium component /
- BP neural network /
- feature extraction /
- pattern recognition
-
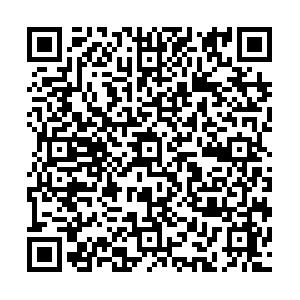
计量
- 文章访问数: 2290
- HTML全文浏览量: 110
- PDF下载量: 449
- 被引次数: 0